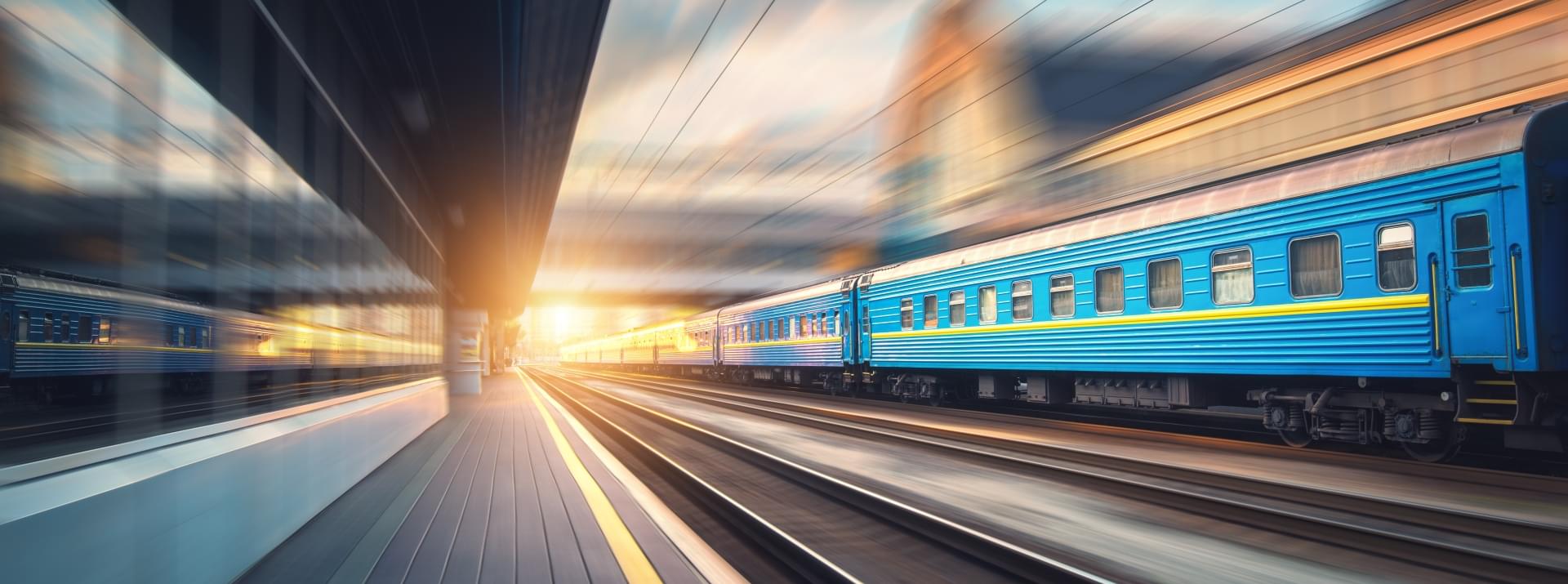
Since 2010, the European Union Agency for Railways (ERA) has accepted the stochastic Monte Carlo method in principle as a viable option for calculating ETCS emergency braking curves. However, the method also offers considerable potential for modeling the mutual relationships within braking systems. If control loops of a system are integrated into the method, it can allow more precise calculation of braking curves, which means that the same level of emergency braking safety could be achieved by applying a lower margin in the form of a higher Kdry_rst value. This would provide opportunities for reducing train headways and thus increasing on-track throughput – without in any way compromising safety. But to bring this potential real-world benefit “to the track” before the impending launch of ATO, it is important to clarify how best to integrate K (correction factor) values computed using the Monte Carlo method into the prevailing safety certification.
Contact
80809 München
Deutschland - Germany
carina.smid@knorr-bremse.com
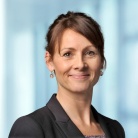
1 Quo vadis, Monte Carlo method?
Luxury yachts, a spectacular Formula One race, casinos… those are the three things most people will think of as soon as they hear the words “Monte Carlo”. But people familiar with probability theory may also think of a stochastic method of computation named after the city-state and used to describe a lengthy series of consecutive random experiments. In theory, these random experiments could be carried out in the real world by rolling dice – but this would, of course, take far too long, which is why computer processors are generally used to generate the random numbers.
The method is extremely useful for calculating statistical averages and deviations. In 2006, UIC submitted a proposal for using the method to calculate ETCS emergency braking curves [1]; four years later, it was accepted as a viable option by the European Union Agency for Railways (ERA) [2]. But the method could also be useful not just for analyzing failure probabilities and performance variations at one hierarchical level in a braking system, but for contributing to the overall design of the braking system, as part of its control loop. This would make it possible first, to draw conclusions about the requisite operational ETCS braking curves at this very early stage in the development process, and second, to calculate the braking curves themselves more precisely.
Abstract
Since 2010, the European Union Agency for Railways (ERA) has accepted the stochastic Monte Carlo method in principle as a viable option for calculating ETCS emergency braking curves. However, the method also offers considerable potential for modeling the mutual relationships within braking systems. If control loops of a system are integrated into the method, it can allow more precise calculation of braking curves, which means that the same level of emergency braking safety could be achieved by applying a lower margin in the form of a higher Kdry_rst value. This would provide opportunities for reducing train headways and thus increasing on-track throughput – without in any way compromising safety. But to bring this potential real-world benefit “to the track” before the impending launch of ATO, it is important to clarify how best to integrate K (correction factor) values computed using the Monte Carlo method into the prevailing safety certification.
2 How the methodology has been used to date
Applied to rail vehicle braking systems, the Monte Carlo method is capable of describing the statistical distribution of braking-related properties, as well as their impact on braking performance. At the same time, it takes account of failure and deviation probabilities in parameters that influence braking performance.
The current starting point is a hierarchical model that mathematically models the effects of a series of parameters. Essentially, these are the parameters that determine the braking system’s architecture. Typical examples include selective control of railcar or bogie brakes; the distribution of the characteristic values of individual braking system components (cylinder pressures, linkage performance ratios, friction coefficients); the reliability and probability of failure of individual system components; the interdependencies between system components; and individual braking system functions. Typical of the latter would be, for example, what is known as high/low braking in the high-speed range.
Because the model links braking-related properties with their statistically occurring probabilities, it also raises the possibility of an additional application: the insertion of arbitrary, random values for these properties in order to simulate a braking system’s random behavior [3].
3 Incorporating braking system control loops into the Monte Carlo method
The same principle can also be applied to the interdependencies (reciprocal interactions) within the braking system. For example, to determine whether one component’s deviation from parameters or actual failure will have a functional impact on other components.
In this case, the starting point is generated by the Monte Carlo model itself, which “randomly suggests” an arbitrary state that deviates from the desired (target) state. This arbitrary state is then tuned, just as it would be in a real-world braking system. Finally, the tuned and stabilized end-state is stored. This “dice throw” or control loop (Fig. 1) is repeated for something in the order of 10 to 100 billion compute cycles, until the correction factors for all requisite confidence levels (EBCLs) have been calculated.
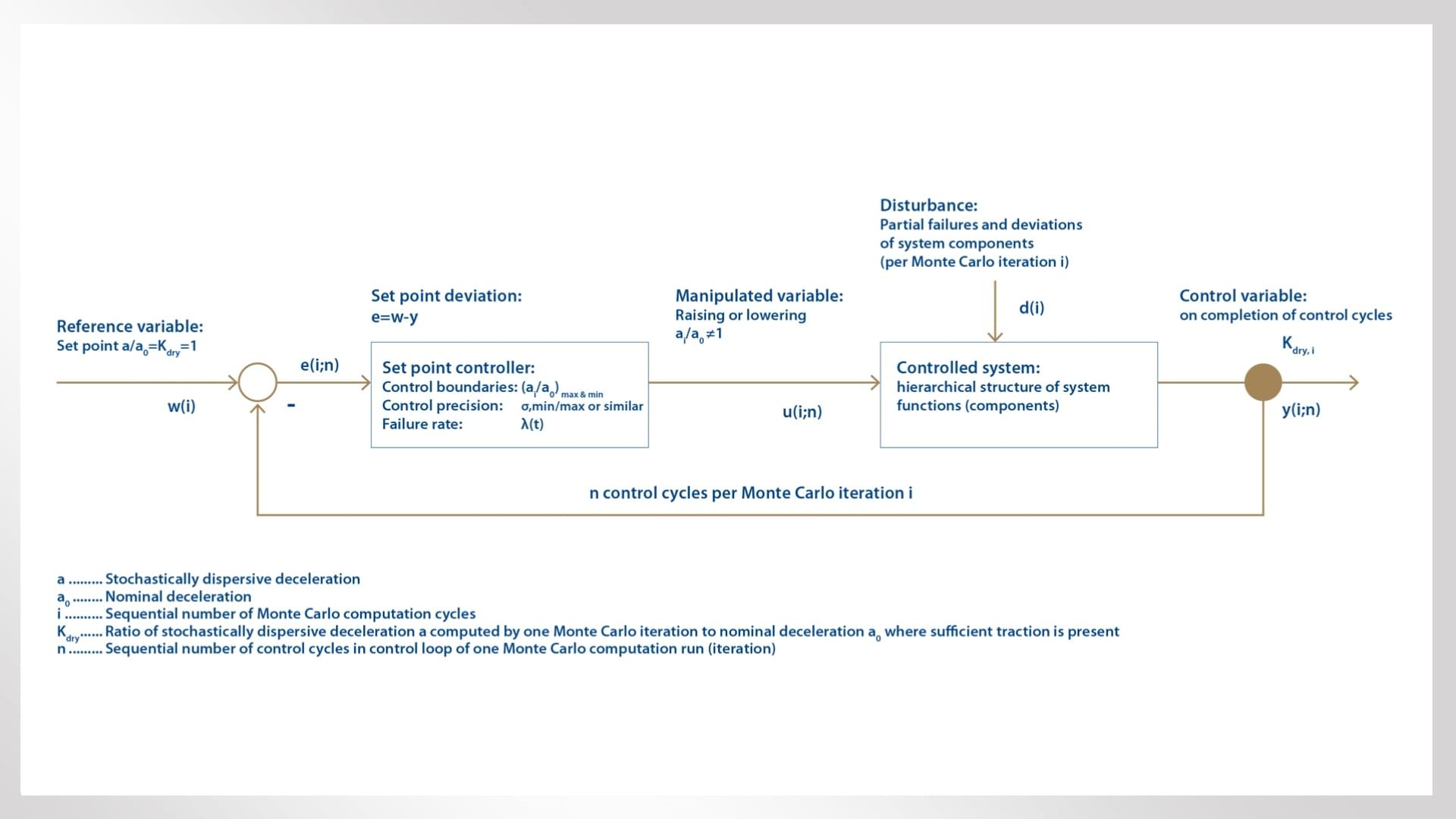
In the same way, a Monte Carlo simulation can now calculate the vehicle- or train-specific Kdry_rst correction factor for almost all conceivable component failures (as well as combinations thereof). This is calculated as a function of speed for the various EBCLs (Emergency Brake Confidence Levels) required in operation, and correlates the deceleration achieved by a braking system in a specific state with the nominal deceleration. As they did previously, Kdry_rst values continue to provide the baseline data required by the ETCS onboard unit to calculate operational braking curves – but these values are not based on formally defined tolerances. Instead, they are calculated using the Monte Carlo method as a function of the probabilities that these braking system states will actually occur.
To understand the overall context, it is important to remember that, as per the UIC proposal made in 2006, the Monte Carlo method evaluates the probabilities that braking system components will fail against the braking performance that can be expected, even if it does not provide an evaluation of the formally attainable safety integrity. But the results do now appear to quantify braking performance during the braking system design process, so that a train could be assigned well-founded safety margins. If conventionally controlled braking systems are extended to include control loops, which are then also taken into account in the Monte Carlo simulation, we may expect to see lower mandatory safety margins – and correspondingly “better” EBCL curves – in anticipation of denser rail traffic (Fig. 2).
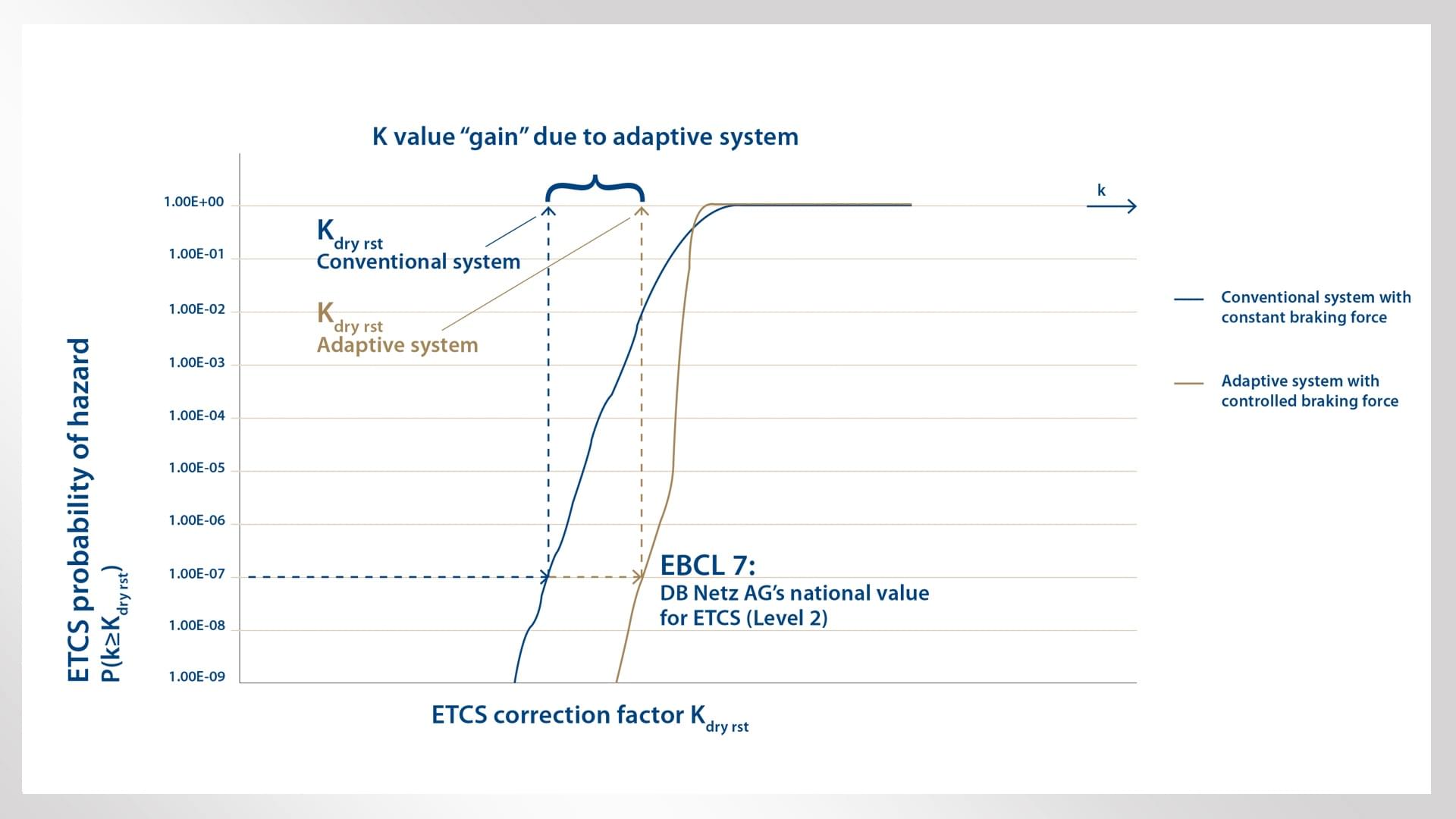
4 Productive application of the method in braking system design
This approach, which now takes account of the interdependencies between the individual braking system components, generates attractive added value in multiple respects. First, Monte Carlo-generated Kdry_rst values would help developers put together modular design concepts where they were previously limited to a specific, defined braking system architecture. And second, the Monte Carlo method could help them calculate dynamic braking behavior – and thus explicitly quantify the extent to which the assumed failure of an arbitrary system component could be offset by another of the train’s onboard braking functions, thereby enhancing operational resilience.
Conversely, the more precisely calculated braking curves mean that the same EBCL could now be achieved by applying a lower “buffer” in the form of a higher Kdry_rst value. Once the resilience of this approach is validated by the relevant safety tests, trains could run at shorter intervals without compromising safety, and thus increase the throughput of a given section of track.
5 Overall context and outlook
At this point, the method is a direct fit with the objectives of the Reproducible Braking Distance (RBD) project. By treating a braking system’s deceleration control, wheel slide protection and traction management as an integrated whole, RBD aims to significantly reduce the variance and divergence of braking distances, even in adverse conditions. On dry rails, and above all due to the reduced braking distance variance, computed simulations have shown in some cases significant ranges of theoretical reductions in train headways: 9%–19% for metro trains, 9%–16% for suburban trains, 1.5%–4% for multiple units on regional lines, and up to 20% for (Chinese) high-speed trains (Table 1) [4].
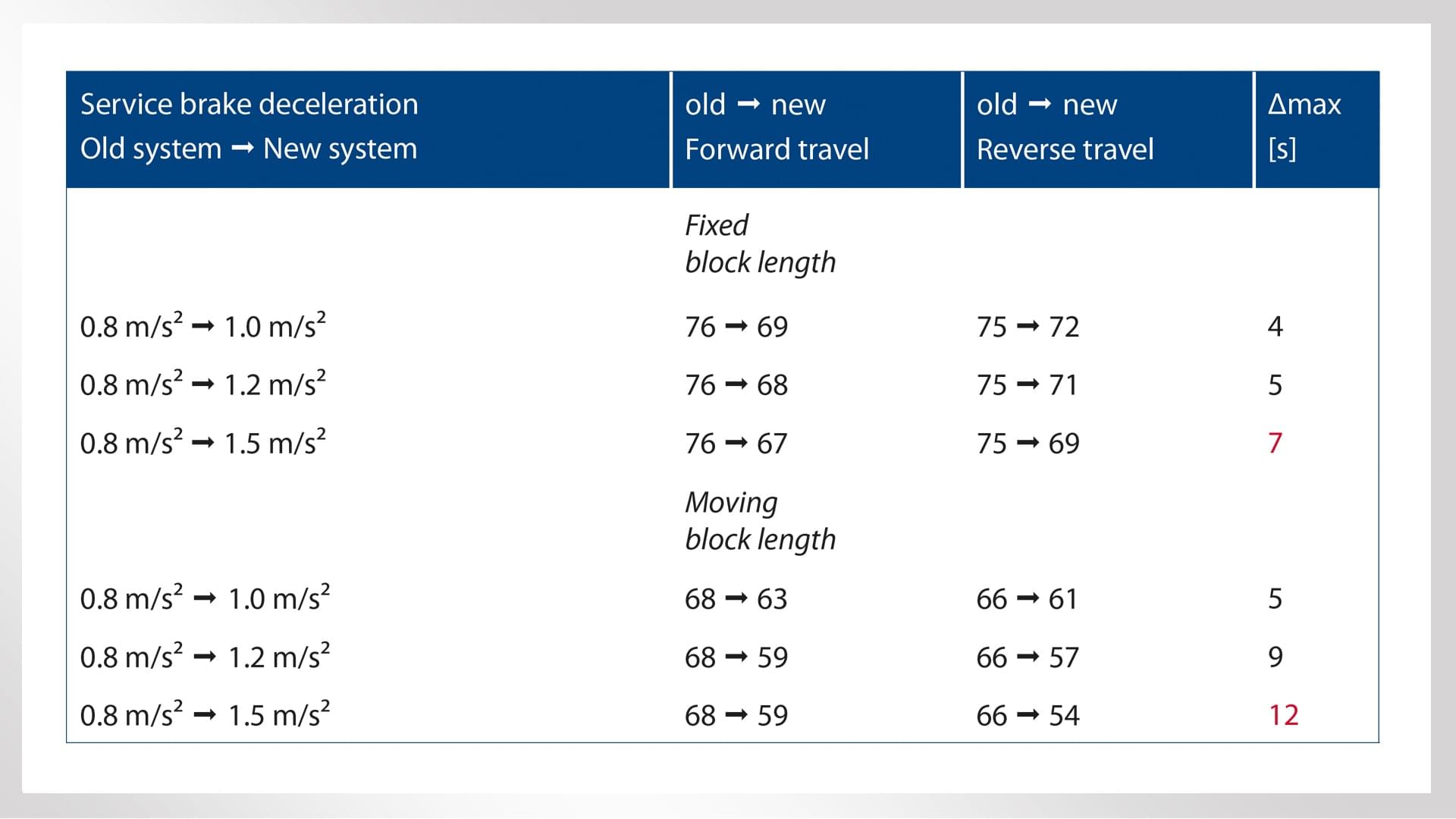
This approach also significantly reduces the Kwet value as an ETCS correction factor in wet rail conditions. In the case of conventional braking systems, deceleration must be reduced by up to half to ensure safe operation – but if the Kwet factor is enhanced by RBD, it is sufficient to reduce deceleration by just one third [4]. This suggests that the Monte Carlo method could close the proverbial circle by integrating individual brake functions and their respective reliability into the RBD concept.
But first, to put the potential real-world benefits of this approach “on the rails” before the impending launch of ATO, it is important to clarify how best to integrate the K (correction factor) values computed using the Monte Carlo method into the currently prevailing safety tests.
Author: Dipl-Ing. Ernst Hohmann
References
[1] UIC B 126/DT414: Braking Questions – Methodology for the safety margin calculation of the emergency brake intervention curve for trains operated by ETCS/ERTMS, June 2006.
[2] Calcul de facteurs de correction pour utilisation comme données d’entrée ERTMS Référence affaire : n° 09-0164, Référence : P/CIM ESF2/2010-0885, Version 2 du 28/04/2010.
[3] Horn, S.; Pavlović, O.: Chancen und Möglichkeiten der Monte-Carlo-Methode bei der Bestimmung der ETCS-Bremskurven. ETR September 2017.
[4] Englbrecht, M.; Gremmel, H.; Hohmann, E.; Linke, T.; Höhere Transportkapazitäten auf der Schiene: Simulation zeigt Potenziale für optimierte Auslastung bestehender Infrastruktur. ZEVrail 144 (2020) 8, S. 276-281.